Understanding spatiotemporal variation of water quality is a crucial but challenging task. We turn to big-data collection and use adaptive-path-planning, sampling algorithms and novel drifting sensors.
Urban water bodies are complex environmental systems, which are further complicated by the dynamics of urban run-offs and closely woven human activities. Maintaining clean, healthy and aesthetically pleasing water bodies for recreational activities and potable use requires careful and effective monitoring to facilitate effective management. While modern robotics has started to improve the traditionally labour-intensive measurement process, measuring varying spatiotemporal field remains a challenge today.
The project aims to improve the sampling efficiency by developing informative path planning algorithms to replace naïve path planning in existing robots. Building on top of real-time data collected by a team of connected robots, such as NUSwan, the algorithms learn time-efficient models of the field and use them to plan paths that minimize the overall error of the estimated field. Additionally, we also extend the path-planning algorithm to sample water and estimate the field simultaneously to ensure valid samples of interest are collected.
The project also integrates cost-effective smart sensors and combines their operations with industrial standard sensors to form a heterogenous monitoring system for big-data collection in the reservoirs. The system consists of NUSwan, drifters and static nodes to collectively improve the monitoring efficiency and accuracy.
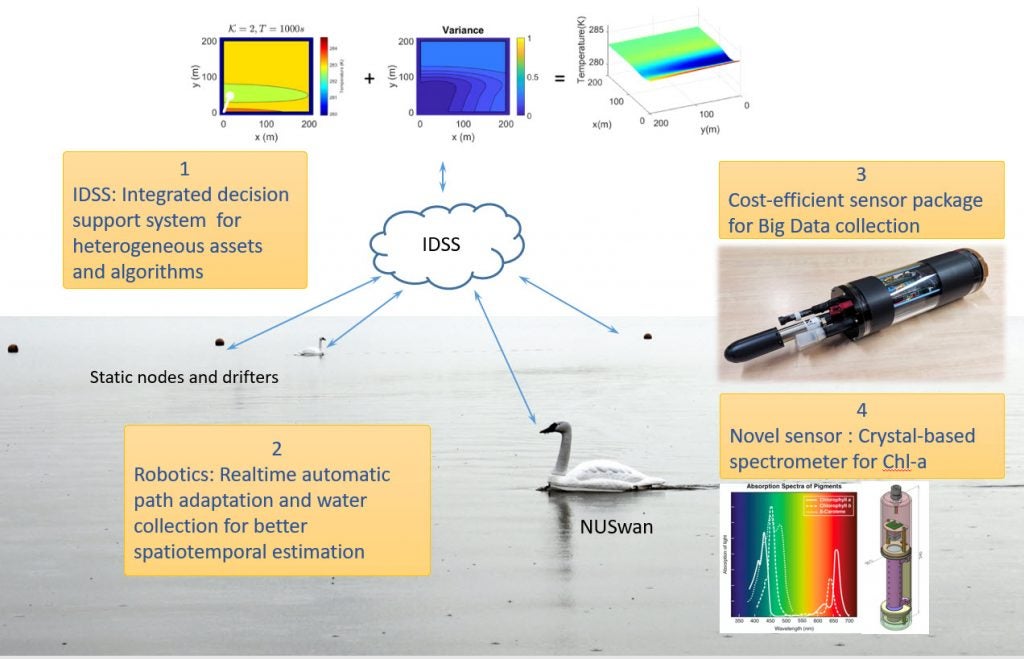